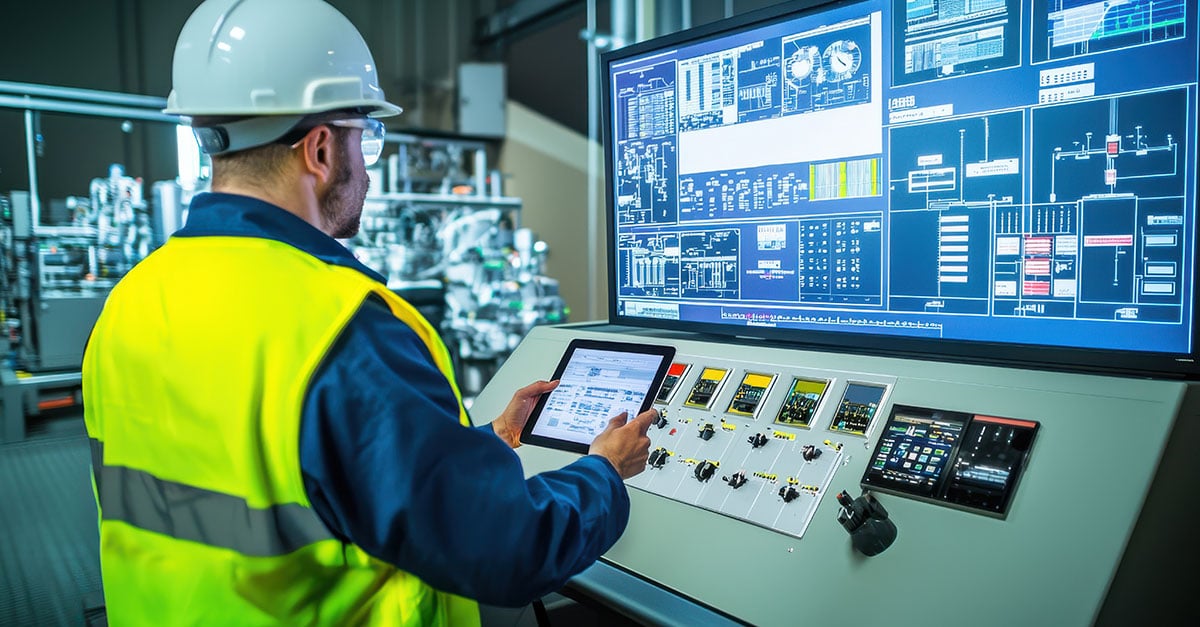
Data Integration - What are the benefits?

Unify machine data structures, combine machine data with other data sources in real time, create standardized data models.
These are the main challenges for Industrial companies to build a ‘digital shopfloor’, achieve a complete and consistent view of operations and performances, and building actionable information supporting a truly data-driven company.
- Data Integration, what it is and how to do it
- Purpose and benefit of IoT Data Integration
- IoT Data Integration best practices
- IoT data pipelines
- Plug & Play IoT Data Integration and Interoperability with Alleantia IoT Edge
- Why IoT Data Integration and Interoperability is so easy with Alleantia IoT Edge software?
IoT Data Integration, what it is and how to do it
Data Integration is the process of cleaning, combining, consolidating and coordinating data from different sources and systems to obtain a complete information set. IoT Data Integration process focuses on the machines and shopfloor data, which present specific challenges: large number of sources, variety of languages and data sets, data volumes, updating speed, correlation with contextual information on processes and operations.
IoT Data Integration goal is creating a reliable and consistent shopfloor information stream, for supporting many data analysis and data-driven processes with clean, complete, actionable information.
Processes include, but are not limited to: production management, predictive and prescriptive maintenance, predictive quality, shopfloor operations management, performance reporting.
Data Integration goes hand in hand with the concept of Data Interoperability, encompassing the transfer and consolidation of data within and between data stores, applications and organizations.
Interoperability is very challenging in the shopfloor environment, given the wide variety of machines' communication protocols, data sets and data models generated by production systems, sensors and operators. Moreover, in a rapidly changing application environment, interoperability is essential to avoid building data silos, whereas machine data must be easily available to a variety of applications, legacy and new, that industrial enterprises have and will build to optimize their operations and creating new ways to exploit the rich information treasury hidden in their shopfloors.
An IoT Data Integration and Interoperability layer should then be present to translate and combine data from different sources, adding contextual information necessary for the use of data by the data-consuming applications, and provide a unified data view.
This objective is hampered by several barriers, such as:
- accessing data from heterogeneous devices and systems
- management of non-integrated OT and IT systems
- complexity and effectiveness of off-line data correlation.
- complexity to scale IoT solutions
With incomplete and un-synchronized data, heterogeneous information, sparse sources, ensuring data consistency becomes a nightmare, as the obtained information only partially reconstruct the course of a process. Data costs money: having a lot of inconsistent data means investing data and process engineers’ time in numerous and endless data collection and reconstruction activities. Finally, it is increasingly common to collect data that will reveal useless and never be used.
Purpose and benefits of IoT data integration
Data integration eliminates the need to manually acquire data from business processes and reduces the risk of errors and subsequent analysis through real-time data.
This simplifies data preparation and avoids having to start over for each report or application, allowing immediate value to be created.
As such, it improves synergy and interaction of systems, saves time, reduces errors, and produces more useful data.
In short, integrating data in manufacturing brings many benefits such as better management and control of production, increased efficiency and accuracy.
However, to take full advantage of these benefits, an effective and sustainable integration process and supporting solutions must be planned and implemented.
IoT Data Integrations best practices
- Find data sources in the company
These may include machinery, ERP systems, MES, quality control, supply chain management and other data generating systems.
- Define the data to be integrated
Once the data sources have been identified, it is necessary to define which data is to be integrated. This may include data on production, inventories, customer orders, shipments, suppliers and other aspects of manufacturing.
- To integrate data, you have to choose a platform
The platform must connect to different data sources. It must bring information together in one place (data lake). This platform should be able to handle large volumes of data, provide accurate data mapping and offer cleaning, and have powerful data normalizing capabilities, including data cleaning, to ensure accuracy, consistency and reliability.
Once the data has been cleaned and normalized, it can be integrated into a single repository.
- Monitor and maintain the data
After integration, it is important to monitor and maintain them to ensure that they are accurate, consistent and reliable over time. This includes a unified view for their periodic verification, correction of errors and cleaning of unused data.
Besides the key steps, it is important to consider some additional elements that can contribute to the success of data pipelines in the manufacturing sector:
- Data security
Ensuring data security is critical to protecting sensitive and critical business information. This includes implementing access controls, data encryption, monitoring of suspicious activity and compliance with data privacy regulations.
- Scalability and performance
Pipelines must be designed to handle large volumes of data and adapt to the growing needs of the business over time. This means they must be scalable and able to maintain high performance even with increased workload.
- Integration with existing systems
Data pipelines must be integrated with the company's existing systems, such as ERP systems, MES, CRM and others, to ensure a complete and integrated view of activities.
- Process automation
Process automation can help improve operational efficiency and reduce repetitive manual work. This can include the automation of data collection and processing, as well as the automation of data monitoring and maintenance activities.
- Real-time analysis
In the manufacturing sector, it is often important to have access to real-time information to make immediate decisions and react quickly to changes in the operating environment. Data pipelines must be able to support real-time analysis to provide up-to-date and timely information to users.
Adding these elements during the design and implementation of data flows in manufacturing can help companies. This allows them to make the most of their data and gain important competitive advantages.
IoT Data pipelines
Data pipeline tools can work to understand potential future trends through predictive analysis based on contextual data. Using efficient data pipeline tools provides in-depth information that can help the production department streamline its operations.
Data pipeline for production monitoring
Collection of production data from machine sensors, Manufacturing Execution Systems (MES), operator terminals and other monitoring devices.
Normalization of data, detection of anomalies, aggregation of data by time period and calculation of production metrics (e.g. OEE - Overall Equipment Effectiveness).
Data analysis to identify production trends, identify machine downtime, predict production peaks and optimize production planning.
Creation of real-time dashboards to monitor production status, generate reports on production efficiency and identify critical points requiring action.
Data pipeline for predictive maintenance
Collection of condition sensor data, historical maintenance data, machine operating data and other relevant equipment health information.
Data normalization, identification of patterns and anomalies in the data, calculation of equipment health metrics.
Data analysis to identify early signs of machine failure, predict the optimal time to perform preventive maintenance and maximize equipment availability.
Creation of dashboards to monitor equipment status, visualize failure forecasts and plan maintenance activities.
Data pipeline for predictive quality
Collection of condition sensor data, machine operating data, quality measurement (in line and offline) and other relevant equipment health information, machine work performance data (e.g. spindle speed, tools usage, temperature).
Data normalization, identification of patterns and anomalies in the data, quality performance degradation, machine operating data correlation.
Data analysis to identify early signs of quality degradation – before test gate scrap -, predict the trend and time to stop production before scrap ratio increases, perform preventive maintenance and maximize production yield.
Creation of dashboards to monitor quality performances, visualize quality degradation forecasts and plan maintenance activities.
Plug&Play IoT Data Integration and Interoperability with Alleantia IoT Edge
Alleantia IoT Edge software is a complete solution to implement all functions for IoT Data Integration and Interoperability in the shopfloor. Within a no-code very versatile framework, it provides all features to ensure quick and complete data acquisition, built-in data standardization, IoT data integration and secure information distribution, available on many OSs either in bare metal or virtualized environments.
The image below summarizes the functions available in the Alleantia IoT Edge software
Plug&play IoT Data Acquisition and Standardization
The Alleantia machine drivers embed the protocol translation together with standardized data structure, converting raw data from any industrial machinery into a common syntax and documented data structure (Gateway ID – Device ID – Variable ID). We make freely available through our Library of Things 5000+ machine drivers, and continuously expanding. The driver editors enable customers, integrators and machine builders to easily create and manage new machine drivers.
Data Models Standardization
Machine drivers can include, beyond the ‘raw’ machine data model, process-driven data models to convert machine data structures into standardized data models, using the ‘virtual variable’ methods that not only can map single variables, but also support math and logical operators to create new variables. We call these ‘Extended Machine Drivers’. For instance, we created – inspired by UMATI Data Models, but also with inputs from our MES application partners – Extended Machine Drivers for machine tools, with a standardized semantic model supporting production process management and MES integration. This model enables customers to use the same input and output data sets (including customer-defined data) to exchange production-related data irrespective of machine types. Moreover, we are building other extended models to support machine data analysis. Customers can freely create their own data models and data mapping logics using the machined driver editors.
Contextual Data Correlation
Through the 20+ APIs available in the IoT Edge software, applications can not only exchange data with machines, but also with the IT Edge software itself, using ‘virtual variables’ in the Extended Machine Drivers. This means that is possible to correlate - real time – machine data with process data, not requiring non-scalable changes in machine programming e.g. adding production order information in the ISO program or creating ‘dummy registers’ in a PLC to host MES information not actually used by machine operations. Using the Extended Machine Drivers and the API configuration, applications can update the IoT Edge software drivers, whereas such information will be associated with machine data in real time and continuously. This will generate complete and standardized datasets that will provide fully consistent machine information.
Data Distribution
The Alleantia IoT Edge software implement a ‘broadcasting’ model, where any of the available API can be configured separately to distribute the ‘IoT Standard Information’ to multiple applications at the same time, with fine-grained setups ensuring robustness, reliability, resilience and security.
Why IoT Data integration and Interoperability is so easy with Alleantia IoT Edge software ?
✅ +5000 MACHINE DRIVERS ready to use - Support for a wide range of CNC, PLC, sensors for plug&play protocol translation, data conversion and processing
✅ STANDARDIZED DATA MODEL: Extended Machine Drivers support standardized data models, either provided by Alleantia following UMATI guidelines, complemented by field-tested feedbacks with customers and application partners
✅ CONTEXTUAL DATA INTEGRATION: 20+ API connectivity with SaaS/Paas applications on premise and on cloud, to add context information to machine data in real time.
✅ NO-CODE DATA DISTRIBUTION: +20 APIs available, configurable independently, for bi-directional data exchange with SaaS/Paas/Applications and services, on premise and on cloud.
Alleantia links your data, from any system, in any format →